Predictive Model for Optimizing Collections Strategy
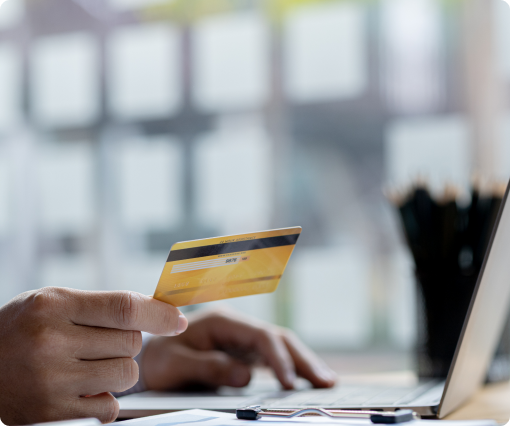
Overview
A leading financial institution sought to enhance its collections strategy by identifying customers most likely to repay outstanding amounts. The goal was to improve collections efficiency and maximize revenue recovery.
Solution
- Predictive Modeling: Developed a probability of default (PD) model using internal, CIBIL, and collections data to predict whether customers in Bucket 1 would transition to Bucket 0 in the next month.
- Risk Segmentation: Derived five PD risk bands (from very low to very high) using the Weight of Evidence (WOE) and logistic binning approach.
- Strategic Insights: Combined risk bands with vintage analysis to assess portfolio performance over time, enabling the collections team to target high-potential customers effectively.
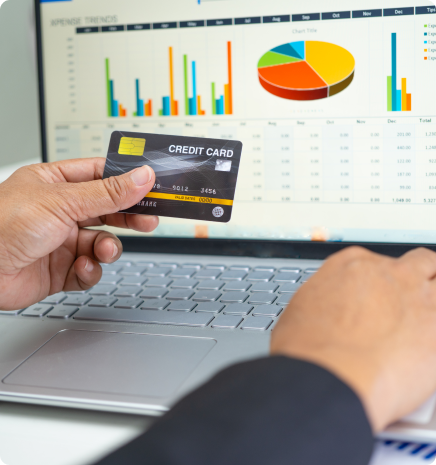
Impacts
Improved Collection Efficiency – Enabled a data-driven strategy to prioritize customers most likely to repay, increasing recovery rates.
Optimized Revenue Generation – Enhanced segmentation allowed better allocation of collection resources, improving overall revenue from outstanding payments.